Health research is a global undertaking with worldwide significance. Research funders need to understand the broader funding landscape to shape their research strategies and ensure the global research ecosystem functions efficiently. Yet, systematic analyses of research funding exist only for very few disciplines. We have supported the first quantitative analysis of mental health research funding on a global scale,[1] working together with the International Alliance of Mental Health Research Funders (IAMHRF) and Digital Science and building on work by the charity MQ: Transforming mental health.
Why analyse global research funding for a specific field of research?
By setting funding levels and designing funding programmes, policy makers and funders directly influence the direction of research. Grants data can provide a detailed understanding of funding flows for a particular area. This allows the detection of gaps in the funding landscape and provides a robust platform from which to ask probing questions about appropriate distribution of funds and lead conversations about collaborations between funders. Measuring funding levels also plays an important role in monitoring funding commitments for a particular area and in assessing returns on investment.
While data alone does not hold all the answers, it is increasingly seen as an essential resource for developing and coordinating funding strategies. For example, the IAMHRF Global Study on Mental Health Research Funding points to stark inequalities, most notably across regions, that – even if not fully unexpected – gain significant weight when rigorously quantified and visualised.
Where to start
When analysing the funding landscape, an important first step is to define the scope of the analysis and the questions stakeholders want to ask. Delineating the funding of a specific field of research from the wider body of related research is an intricate task, and grey areas and uncertainties will likely remain. Building on existing taxonomies and consulting with experts and stakeholders ensures buy-in and alignment with important reference datasets such as burden of disease statistics. Taxonomies can also be derived from data directly, for example using tools that assess the similarity between different texts to derive clusters, or tools that are based on citation links of publications.
In our particular example, mental health research is challenging to define because it is closely related to neurological research. Yet, work by the charity MQ: Transforming mental health has demonstrated that it is possible to define usable inclusion and exclusion criteria as well as sub-fields for the field of mental health research.[2] The IAMHRF Global Study on Mental Health Research Funding builds on this foundation.
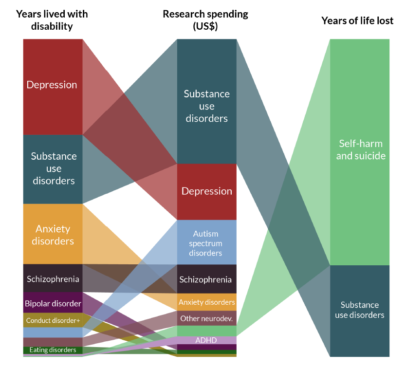
What can funding data tell us?
Research funders commonly hold a record of their funded projects and increasingly share information on their portfolio publicly. Available data points typically include a short description of the project, information about the funding organisation, the hosting institution and the principal investigators, funding amounts, and the project timeframe, as well as automated classification using one or several standardised systems such as Field of Research, the Health Research Classification System, or Medical Subject Headings. Unfortunately, as found in the IAMHRF Global Study on Mental Health Research Funding, publicly available funding data still has important gaps, including large parts of philanthropic and practically all industry funding. More detailed data is often held by funders – but not usually shared outside the organisation due to confidentiality. However, this could in principle be used for classification within the funders’ protected environments.
Project abstracts are usually sufficient to classify projects according to disease areas, which helps identify neglected areas. The IAMHRF Global Study on Mental Health Research Funding revealed that relatively little research had addressed the issues of suicide and self-harm, eating, conduct and personality disorders.
Projects can also be mapped onto the translational research pathway, which gives insights into the level of funding provided at different stages, from basic research, research into aetiology and prevention, to the development of treatments and health services research. Geographical data can be used to assess where funding flows, though information about subcontracted institutions may be limited. Information on the research teams (e.g. discipline, demographics) is limited in public grants databases, but could in principle be derived using bibliometric approaches. Likewise, details on study participants (e.g. age, gender, race) are not readily available, but can to some degree be inferred from project abstracts. This was done in the IAMHRF Global Study on Mental Health Research Funding, showing that younger and older age groups receive smaller shares of funding.
Manual classification vs. natural language processing
Before the widespread use of machine learning and natural language processing, landscape analyses were resource intensive. Potentially relevant grants were identified using funder profile, funding stream, or keyword searches. Human coders then had to read titles and abstracts of each project to assign any classifications for analysis. This required working closely with participating organisations and training a large network of coders in standardised coding rules. While it is helpful to deeply engage funders, the associated costs can also present a barrier to participation and limit the coverage of the resulting dataset.
Machine learning can identify and classify relevant grants in an automated way, allowing large amounts of data to be processed quickly. The accuracy of automated classification depends on many factors, including the quality of the available data (i.e. how clearly does the project description articulate the focus of the funded research) and the sophistication and training of the classification tools. It is important to note that manual classification is not free from error, and ambiguities and grey areas mean that different human coders may disagree on the coding for a particular grant.
The IAMHRF Global Study on Mental Health Research Funding fully relied on pre-existing automated classification tools implemented on the Dimensions database and as well as bespoke tools made available by MQ: Transforming mental health.2 Testing small samples manually showed that accuracy was acceptable for a first scoping study of this kind, although there is clearly room for improvement. A recent study has found automated approaches can even outperform human coding.[3]
Final thoughts
Research funding analyses provide important insights that can help to define and coordinate funding strategies within particular fields of research. They are much facilitated by recent trends in data sharing and harmonisation, alongside advances in automated processing of text data, which have substantially dropped the cost. It is critical to involve stakeholders early in the process to ensure analyses are useful and to maximise data quality and completeness. The IAMHRF Global Study on Mental Health Research Funding has demonstrated that it is feasible and useful to gather data about mental health research funding on a global scale – a concerted effort by the global community of mental health research funders is now needed to build a longer-term solution for repeated and refined analyses.
The IAMHRF Global Study on Mental Health Research Funding is available here.
[1] Woelbert E., Lundell-Smith, K., White, R. and Kemmer, D.(2020). Accounting for mental health research funding: developing a quantitative baseline of global investments. The Lancet Psychiatry. https://www.thelancet.com/journals/lanpsy/article/PIIS2215-0366(20)30469-7/fulltext
Woelbert, E., White, R., Lundell-Smith, K. Grant, J. and Kemmer, D. (2020). The Inequities of Mental Health Research. International Alliance of Mental Health Research Funders. https://digitalscience.figshare.com/articles/report/The_Inequities_of_Mental_Health_Research_IAMHRF_/13055897
[2] Woelbert, E., Kirtley, A., Balmer, N., & Dix, S. (2019). How much is spent on mental health research. Developing a system for categorising grant funding in the UK. The Lancet Psychiatry. https://doi.org/10.1016/S2215-0366(19)30033-1
[3] Goh, Y.C., Cai, X.Q., Theseira, W. et al. Evaluating human versus machine learning performance in classifying research abstracts. Scientometrics (2020). https://doi.org/10.1007/s11192-020-03614-2