Behind the hefty walls of public institutions and governmental bureaucracy hides a fascinating yet Kafkaesque tango dance between public policy and generative AI. Science and technology policies have actively championed the development of fundamental AI knowledge and infrastructure, which served as the building blocks for public and private research to advance the field into what it is today. Equally significant, public policy investments in higher education have been vital in nurturing a pool of highly specialised AI talent from universities, enriching the sector with skilled professionals. On the other hand, the distinctive nature of new AI data-driven businesses is pushing the limits of traditional competition policy frameworks and ruffling the feathers of regulators in competition authorities. Additionally, the potential for automation and its profound impact on social welfare and inequality are causing grave concern among labour and social policy officials.
Amid the exciting tango between generative AI and public policy, there lies an additional dimension: applying generative AI tools within the realm of public policy itself.
At Technopolis Group, we know that we must maximise the benefits of technological advancements while minimising their potential risks. We strive to maintain our reputation as leaders in providing original analysis, robust data and evidence-based policy recommendations while innovating with disruptive technologies like generative AI. These two forces are not always aligned: as the field moves fast with an avalanche of new models and uses affecting almost all aspects of our lives, ensuring responsible, ethical, and high-quality use of existing tools can come at the expense of fast absorption. “Moving fast and breaking things” has proved successful in speeding up some technological applications, but often with worrying social consequences and breaks in public trust.
The opportunities for improving public policy with generative AI are immense, as are the risks and the scope for breaks in public trust. Therefore, at Technopolis, we are focused on tackling this friction and enabling human-centred generative AI applications that are responsible, accountable, and transparent. We learn from standard-setting organisations, such as the OECD AI principles, the European Union’s AI Act, the Unesco Agreement of AI Ethics, and the UK’s Pro-innovation approach to AI regulation. However, our own internal principles and journey are hands-on because we are, ultimately and proudly, public policy plumbers.1
This publication will take you through part of our ongoing journey in developing applications of generative AI in public policy contexts. We will delve into the broad spectrum of challenges and opportunities we are encountering and share parts of our strategy. Yes, a bit of a sales pitch here 😉 – but we are excited about our unique approach, centred on responsible and transparent AI use. Our applications harness the synergy between Technopolis’s deep pool of human intelligence, refined through decades of qualitative policy expertise, and the energy of our data scientists, who are deeply committed to improving public policy. We will cover the following topics: first, a summary of opportunities and ongoing applications, followed by our approach to fully harnessing the potential of these tools. Our approach considers data privacy, transparency concerns, and the interplay between colleagues of diverse academic and professional backgrounds.
Strategy And Principles
When Technopolis launched its internal Data Science Unit, OpenAI’s ChatGPT and other generative AI tools had just begun to sweep the world off its feet. This technology completely disrupted the original business plan for the unit. Its members and Technopolis’ leadership understood this is a game-changing technology and required a set of principles guiding adoption among the whole company (regardless of data science skills, thematic specialisation, or seniority).
We developed a simple set of orientating principles, considering they will always face the trade-off between generality and usefulness. Our clients know us for providing original, independent, and accountable public policy analysis and recommendations. Being in the business of human intelligence, our principles guiding the use of generative AI tools must entirely align with these values and enhance – not replace – our core strengths. With the field moving at a fast pace, being too prescriptive risks becoming quickly outdated. On the other hand, being too general and abstract can be hard to understand and apply to our daily applications. Therefore, our principles are straightforward, and our colleagues know we will constantly update them: simple and live principles.
Figure 1. Technopolis Group (TG) Generative AI principles (Hower through each principle for more details)
Source: Technopolis Group
Navigating Trade-Offs
We can pinpoint our primary risks and challenges by examining the critical trade-offs between our principles and the seamless use of generative AI. Certain precautions are obvious: inputting confidential data into large language models without care or failing to fact-check the outputs clearly violates our protocols. We consistently emphasise these red lines to our colleagues across all levels of seniority. Other trade-offs are more complex and case-specific, requiring more profound thought and team discussion, such as potential biases and ethical consequences of using specific outputs. Moreover, we foster discussions about good practices by compelling colleagues to own their usage and being transparent about all of their practices with generative AI. We believe this approach ensures higher quality use, but we must accept that a trade-off exists between responsible use and speedy adoption.
One trade-off of significant concern that we still do not fully grasp relates to the short-term productivity gains vs long-term effects of generative AI. We strive for colleagues of all seniority levels to think of replacement vs reinstatement effects: we can use generative AI to automate simple tasks or enhance our colleagues’ skills. Some technological innovations have obvious distinctive effects (e.g. autonomous checkout machines mostly automate labour, whereas robotic-assisted surgery is mostly reinstating). Generative AI can have both, making it harder to navigate. The primary danger for our company is that by automating more straightforward tasks, often performed by junior colleagues, we may find short-term productivity gains but deprive junior colleagues of the necessary learning-by-doing they need to grow. 2
Besides the long-term impacts of automation on our teams and broader society, we must give more profound thought to how generative AI affects the generation of new insights. Generative AI relies on fresh content to maintain its usefulness, having traditionally sourced vast amounts of text from the web. However, the rising popularity of tools like ChatGPT marks a notable shift in online behaviour, often resulting in decreased traffic to traditional websites. This trend could inadvertently impact these websites’ motivation to produce fresh content. The ripple effect is significant: with less original material available, the resources for updating and training generative AI tools become scarce. This potential content drought could challenge the continuous advancement and effectiveness of AI technologies, prompting a need for innovative solutions (see Figure 2 for some worrying trends between ChatGPT and Stackoverflow – one of the primary sources of training data for coding text). Our team proactively acknowledges this risk and consistently reminds our colleagues of these tools’ primary goal: to augment, not replace, skills. We emphasise the importance of leveraging our critical competitive advantage — creative human intelligence, which must remain the primary source of our original content.
Figure 2. Google Search Trends and Estimated ChatGPT Site Visits
Source: Google Trends (StackOverflow topical search and ChatGPT entity) and SimilarWeb for estimated site visits
The Opportunities: Is It All About Speed And Costs?
The number of reports about applications of generative AI in the public sector is booming. Without a doubt, generative AI enables organisations to do the same at a higher speed and lower costs, which is somewhat dull. Cost-savings are productivity-enhancing, but the tool’s potential is much greater.
Generative AI is poised to revolutionise operations within government sectors by enhancing a range of services. It can streamline programme management through content summarisation and synthesis, making complex information more digestible and accessible to both government officials and the public. This advancement promises to facilitate quicker, more informed decision-making processes and improve public service delivery by tailoring them to the specific needs of diverse populations. In the realm of policies and regulation, it distils complex research into concise regulatory summaries and synthesises for standard operating procedures. Infrastructure projects also benefit from its ability to consolidate licensing processes and make them more accessible. In coding and software, generative AI transforms outdated code bases into modern languages, facilitating a smoother transition to current technologies. When it comes to customer engagement, AI-driven chatbots are improving citizen interactions, and they are streamlining processes like license renewals through expert systems that provide advice and point to relevant official sources of information. Content generation through generative AI offers a personalised approach to programme content, legal and regulatory guidance, and emergency instructions, ensuring that government communication is tailored and responsive. This integration of generative AI into government operations underscores its potential to improve efficiency and compliance and provide citizens with more responsive and personalised services.
In a recent example, we deployed our internal Technopolis AI Policy Concierge tool to support our analysts in performing a policy project in 5 months, which took the incumbent three years. In building the S3 policy observatory, we used generative AI to identify relevant topics and tag regional innovation strategies against hundreds of relevant categories. 3 Each strategy can contain over 200 pages, and the project required digesting documents on the hundreds in different languages and from regions with heterogeneous economic specialisations and strategic priorities. We enabled the automated summarisation and tagging of all the documents in a systematic fashion, providing our analysts not only final tags but also a transparent justification linked to the source text of each document. In addition to the large-scale summarisation and classification, we enable our colleagues (and clients) to “speak” to the data, querying individual or groups of documents regardless of language or size. By providing this support, we allow our analysts to specialise in deriving policy insights and recommendations instead of specialising in pure translation and information retrieval. Moreover, by providing justifications and links to the source text of each answer, we prevent hallucinations and can always enable fact-checking, fully exploring synergies between AI and our analysts’ policy experience.
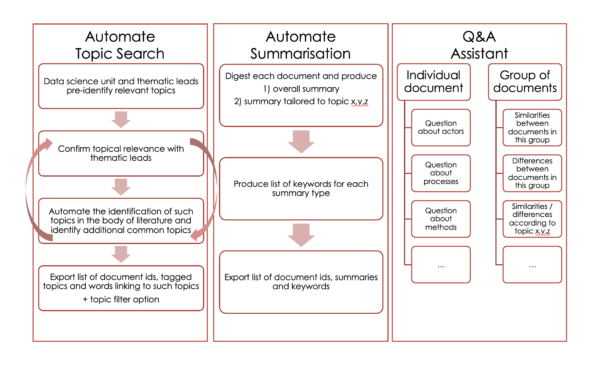
Source: Technopolis-Group
The Technopolis AI Policy Concierge exemplifies how AI enables us to achieve unprecedented levels of speed, quality, and detail in our work. The critical value of generative AI lies in our ability to enhance and fine-tune tools through our extensive policy expertise, distilled from internal documents and project data accumulated over decades and cross-validated by our qualitative experts. It empowers our colleagues to focus on what we excel at and are passionate about — deriving deep insights for public policy. We work more swiftly and with the capacity to process information on a scale and with a degree of detail that was previously unattainable. We develop applications while adhering strictly to our privacy and confidentiality standards, and in case of extremely sensitive information, we can ensure data remains within European servers, thus avoiding the commonplace data transfers to locations like California.
Hidden Costs And Challenges
While the development of AI tools has significant expenses, the hidden costs often pose greater challenges in planning and estimation. The more apparent expenses, such as data scientist work hours, data processing infrastructure, and access to large language models, are of significant importance, but we cannot disregard the hidden costs. These include the time spent explaining the function of the tools to colleagues without data science backgrounds, the additional hours our data scientists need to understand policy nuances, and the significant cost involved in collaboration with qualitative colleagues to maximise the tool’s value (see Figure 4 for a summary). Furthermore, additional expenses are associated with explaining the process and its benefits to clients.
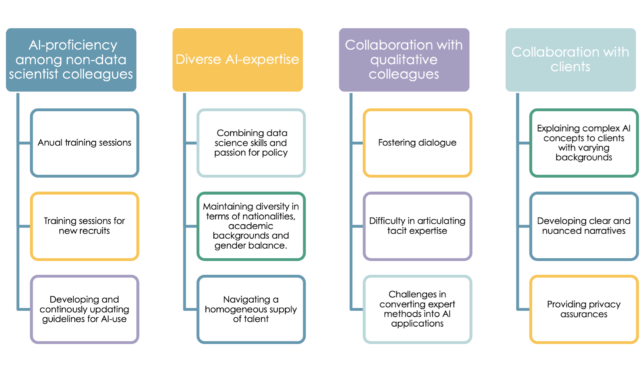
For effective AI integration, colleagues in the policy and research team, possibly with limited data science expertise, must understand the intricacies of AI applications within their work. This requirement is a significant endeavour. In preparing the policy and research team to integrate AI technologies, we prepared formal guidelines and tips for AI use, annual training sessions during “techno days,” and training sessions for new recruits. The challenge in developing guidelines lies in creating a document that is both comprehensive and accessible to a broad audience, not just data scientists. Guidelines for navigating this fast-evolving field should be general yet not overly prescriptive. The document will be a live and continually updated resource, requiring continuous efforts.
Effective use of AI requires thinking beyond the data; thus, our data scientists must be more than data experts. They should understand the intricacies of working for the public cause with a good general understanding of policy issues and specialising in some policy areas. Therefore, we want to remain diverse to avoid funnel/homogenous thinking and always find space to think of the social impacts of our work and the caveats of using distinct types of data. Moreover, diversity within the team may facilitate improved communication with clients, especially given that clients represent a wide variety of fields, nationalities, and cultural backgrounds. However, combining advanced data science skills with a passion for policy and specific policy areas, such as health, science, international development, or sustainability, can be challenging. Nevertheless, we have achieved this so far with a diverse team, including six different nationalities in a team of seven, working from five different countries. Additionally, we have established a good gender balance and diverse academic backgrounds ranging from Economics, Astrophysics, Psychology, and Management sciences. We are deeply committed to this endeavour, yet it poses a significant challenge due to the highly homogeneous supply of talent. Consequently, the time required to achieve this balance in a continuously expanding team also represents a hidden cost.
The AI, designed to mimic human cognition, necessitates a dialogue between data experts and qualitative colleagues who are not directly involved in AI. This interaction ensures a comprehensive understanding of the data and its implications. It also ensures the development of high-quality AI tools and allows cross-validating outputs to maintain expected quality standards. In terms of decision-making processes related to AI implementation, we also spotted a competitive advantage in leveraging the qualitative input from colleagues who contribute their expertise and data. For example, regarding ethics, we use the expertise of qualitative colleagues in the field of AI ethics as their input in shaping ethical considerations. The interaction between the data science and qualitative teams is essential in driving policy recommendations and providing a unique edge in the market. Nevertheless, fostering dialogue with the experts can present heavy challenges. Due to their considerable experience, the methodical nature of their work becomes intuitive, leading to a loss of awareness of their analytical structure. This reliance on tacit knowledge can make it difficult for experts to explain their own processes, complicating the translation of their methods into AI applications.
As a consulting company, our ability to integrate AI into our work is highly affected by our clients’ attitudes toward AI. Client knowledge and receptiveness to AI vary significantly. Clients in some countries/fields share a great enthusiasm for exploring new methods and ideas, demonstrating a willingness to take risks and challenge the boundaries of traditional approaches. However, this is not the same across all countries, and the company often encounters concerns about clarity and a lack of knowledge about AI. We are working on technical implementation, clear communication, nuanced narratives, and privacy assurances to address these challenges. We must be able to explain complex AI concepts to clients with varying backgrounds to build trust and make sure they understand the benefits of using AI. This process requires significant effort and may result in substantial expenses. Additionally, protecting sensitive data is paramount but costly, highlighting an additional challenge in terms of client relations. Conflicts can arise between performance and customer expectations, accentuating the importance of communication and explaining why our services may take longer and be more costly compared to companies using AI without the same transparency and security requirements.
Key Takeaways And The Path Forward
Embracing The Future With Responsibility And Innovation
As we stand at the threshold of a new technological era, generative AI will seamlessly intertwine with the intricacies of public policy. We must acknowledge both the vast potential and the inherent challenges this integration presents. At Technopolis Group, we have embarked on a journey, pioneering the responsible and transparent use of AI in public policy, a path marked by innovation, ethical considerations, and a commitment to enhancing human expertise with technological advancements.
Enhancing Human Intelligence, Not Replacing It
Our central tenet remains the augmentation of human intelligence, not its replacement. By synergising the deep pool of human expertise with the dynamic capabilities of AI, we strive to foster a landscape where informed decision-making and policy formulation benefit from both the nuanced understanding of human intellect and the efficiency of AI. This symbiosis is not without its challenges, requiring us to continually navigate the balance between ethical use and rapid technological adoption.
Navigating the Complex Landscape of AI Integration
The journey of integrating AI into public policy is replete with both opportunities and complexities. The potential for AI to streamline processes, enhance service delivery, and provide tailored solutions is immense. However, this journey also demands a meticulous approach to managing the hidden costs and challenges, such as ensuring our diverse team comprehends the nuances of AI applications and maintaining a balance between data science expertise and policy understanding.
A Commitment to Continuous Learning and Adaptation
The landscape of AI and public policy is ever-evolving, and so is our approach. We commit to a process of continuous learning and adaptation, keeping abreast of the latest developments in AI and responding proactively to the changing needs of public policy. Our journey is one of collaboration, innovation, and firm dedication to the public good as we embrace AI as a transformative tool in the realm of public policy.
Looking Ahead: A Vision of Collaborative Growth
As we look towards the future, our vision is clear – to remain at the forefront of AI integration in public policy while upholding our principles of responsibility, accountability, and transparency. This process involves continuing to develop innovative AI applications and actively engaging in dialogue with our peers, clients, and the wider community to share knowledge, address challenges, and shape the future of public policy in an AI-driven world.
In conclusion, integrating AI into public policy is a journey of balancing innovation with responsibility, enhancing human intelligence with AI, and navigating complex trade-offs. At Technopolis Group, we are committed to leading this journey with our principles, expertise, and vision, shaping a future where AI serves as a catalyst for informed, ethical, and effective public policy.
Authors: Alice Adler Runow and Diogo Machado
[1] In allusion to Duflo, E. (2017). The economist as plumber. American Economic Review, 107(5), 1-26.
[2] Concerned inspired by Johnson, S., & Acemoglu, D. (2023). Power and Progress: Our Thousand-Year Struggle Over Technology and Prosperity. Hachette UK.
[3] See current project output here: https://ec.europa.eu/regional_policy/assets/s3-observatory/index_en.html